This interview with user experience expert Peter W. Szabo charts the progress of UX towards personalisation, a three-step process for perfecting it, and the scale of the possibilities it offers the betting and gaming sector.
User experience has long played a vital role in the success of software development. That’s why a huge emphasis has always been placed on perfecting it – to ensure that users find their experiences more engaging, frictionless and satisfying.
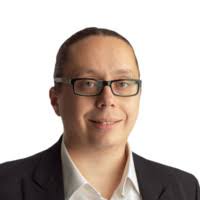
However, today UX designers face a new challenge: meeting the increasing demands from users for personalised experiences that fulfil their specific needs and desires.
“The problem is that the users are vastly different,” Peter W. Szabo explains. “It’s no longer enough to just cluster them into personas or certain other constructs to try to create a few different user journeys. That’s because ultimately, all users want slightly, or not even slightly, different things. So, ideally you would have a unique tailor-made experience and a tailor-made user journey for all of your users. That would be the ideal scenario.”
How one size can fit all
However, getting to this highly personalised future state is easier said than done. For a start, it will require a change of philosophy in how UX is designed. That’s because, according to Peter, current processes can please a majority of users some of the time, but they can’t please absolutely everyone all of the time.
“Currently, the way UX is created is by conducting the research on a subset of users, in other words, observing their behaviour and journey,” Peter says. “The UX designers create the design and then maybe turn into a rapid prototype. Then they test again, create a new design again and so on and so forth. You’re then trying to sell it as a design, which will be good for all users, and to a degree, it will be good for hopefully a large percentage of them. But in reality it will probably not be ideal for any of those users, and a smaller percentage will be dissatisfied with the experience you created because you are treating users equally.”
The solution, Peter believes, can be found within the process of collecting and understanding detailed information about each individual user. And that’s something only feasible by leveraging next-generation technology.
“Users are not the same and they have different needs, expectations, different behavioural patterns, mental models and so forth,” Peter says. “So I believe the future of UX is heading in the direction where we can create a different experience for each and every user. And this is where machine learning comes into the picture.”
Three steps, endless possibilities
Peter expects eCommerce to be among the first industries to fully exploit the potential of machine learning to aid UX personalisation. The key drivers for this are the highly competitive, open nature of the sector, and the direct link between better UX and increased sales.
Peter advocates a three-step process for perfecting machine learning-based UX, as follows:
Data analysis: “You need to gather lots of data from your users, mainly event data,” Peter explains. “So you want to know what sort of events they have during their visit. On an eCommerce platform, for example, this would be things like viewing products, adding products to your cart and purchased products. Then you analyse this and try to explore the patterns in that data.
“At this first stage, you don’t need to worry too much about the correctness of your assumptions or statistical accuracy. You just explore the data and get it ready for step 2 – it’s hard to go much further because usually the data quickly becomes an insanely large data set.”
Data filtering: “To be able to handle the volume, you need to reduce the data in a meaningful way, which enables the solution to scale really well,” Peter says. “I have created a mathematical model, which enables you to basically discard all the junk from the data and only keep the most relevant part, and then transform that into what we call ‘sparse matrices’. Then, on those sparse matrices, which are now much more efficient, you can run a machine learning algorithm.”
Application of machine learning: “These algorithms are enabled by applying recent advancements of machine learning to modern libraries such as PyTorch,” adds Peter. “Then you would run your machine learning model on a GPU or TPU, and this would predict the likelihood of certain events.
For example, you could use this to predict that a specific user would buy certain products and show the products to them, even before they searched for them. Or, if you know that there is a particular product category that they are interested in, you can also show them articles about it. Further, you can even customise your homepage, provide special offers and so on. The possibilities are limitless.”
A winning bet for gaming
The wide variety of potential experiences that users may value and the vast amount of data available, makes the betting and gaming industry an ideal candidate for exploring these opportunities. Indeed, Peter envisages interfaces and preferences automatically focused on a user’s preferred sport, or even their favourite team.
“For example, in football an Arsenal fan would see the app in their colours with matching backgrounds,” he suggests. “They would receive notifications to bet on the next Arsenal games – maybe they’d get suggestions based on their favorite players. All users would start with the same interface but as the events are dispatched, the interface would be customised based on their actions.
“The algorithm would learn their preferences without needing to be set: it just automatically works. The ideal user journey would be to store the event data and then feed it to the machine learning part of the solution. Then it creates a configuration, which will be pushed to the application prompt, and then the user would see a different look and feel for the front-end itself.”
And far from just being an evolution of UX, Peter believes the combination of machine learning and the cloud can spark a radical revolution.
“It could work on mobile, tablets or whatever, because the machine learning runs in the cloud anyway,” Peter concludes. “You could use Amazon’s AWS, or Microsoft Azure Cloud, or Google, or Alibaba or whatever you prefer. Ultimately, I believe that this machine learning and behavioural analysis will rewrite the rules of UX in the next decade. I believe that UX as we know it will radically change.”